Attrition is a real problem for mobile marketers who spend millions to acquire new users only to lose them within days of installing. Attrition (churn) is costly too. Not only are marketers spending to acquire new users, the cost of reengaging them exceeds the initial investment.
Churn analysis is a solution but requires a significant time investment by the marketing team. Essentially, there is no quick or single way to answer as to why users aren’t engaged.
To help marketers reduce churn, Kochava has developed Churn Modeling as an additional event in Analytics to help marketers identify users most likely to churn. Using machine learning, churn modeling identifies patterns exhibited by the users most likely to churn. Once identified, it scores and groups users according to their risk level.
Gain an advantage with churn modeling
The Churn Modeling event (“_PredictedChurn”) is the result of a machine learning model that predicts a user’s likelihood to churn. First, the algorithm “learns” user behaviors that indicate churn based on historical data. This data includes recency, frequency, and trend metrics observed during the first seven days after an install. The resulting model is then applied to new users and predicts which users may churn in the subsequent 30 days.
Users are grouped based on their likelihood to churn (high, medium high, medium low, low). They are also scored with their probability to churn (between 0 and 1), which can be useful for customizing view and filters. The models get updated on a regular basis; if new events are tracked, the models will update to account for any new patterns that emerge in the data.
Retarget with Engagement push notifications
Having Churn Modeling as an event in Analytics directly lends itself to push notifications. Evidence shows that users who are more likely to churn respond positively to push notifications. With Kochava, marketers can identify an audience at risk for churn in Analytics and activate a push campaign for them with the help of a push-notification platform.
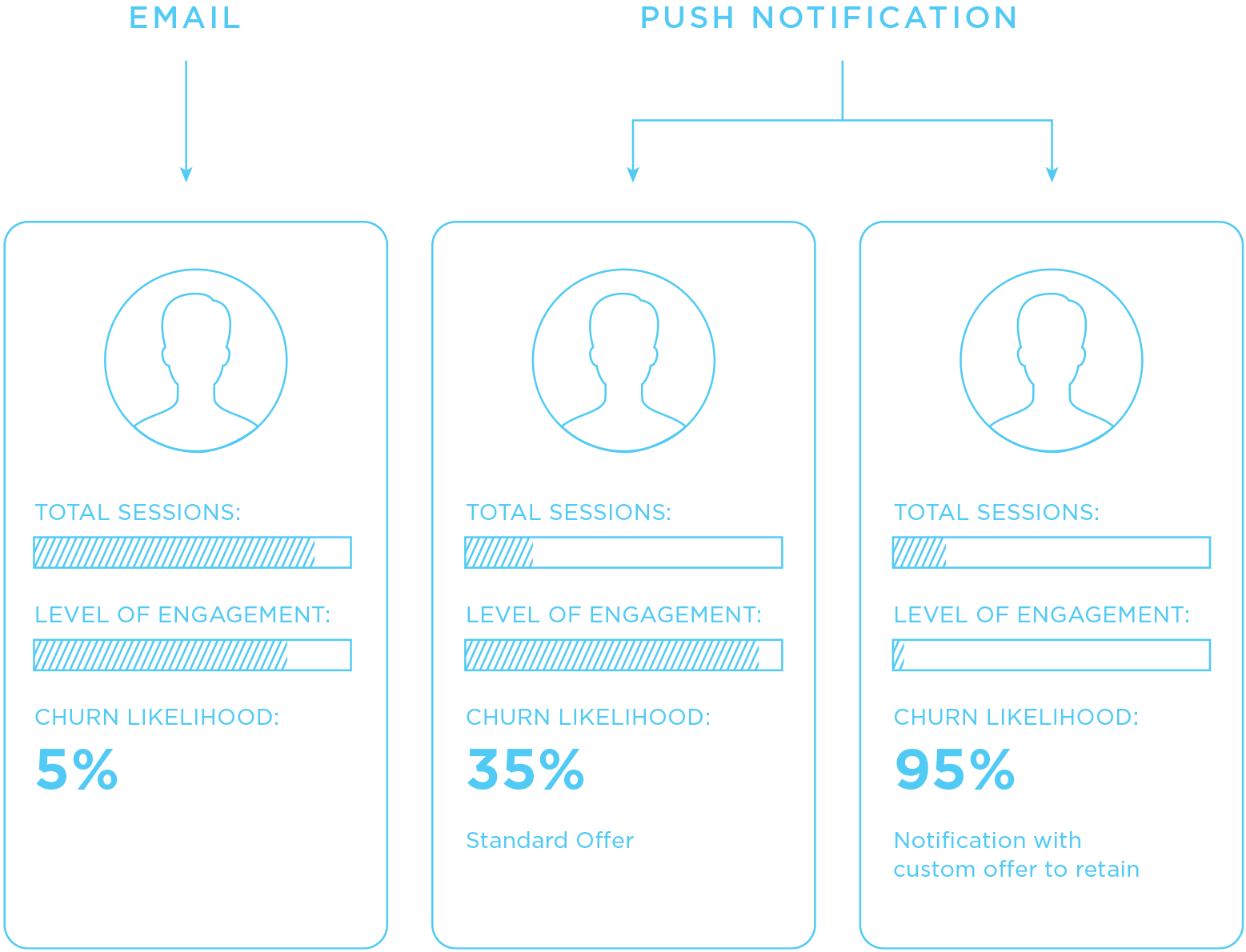
For example, in Analytics, create a cohort of users unlikely to complete a purchase and immediately engage them with an incentive to convert. Select the “Add to Cart” event and the “Predicted churn” event, and exclude users who have already purchased. This will create a bucket of users that have expressed intent to purchase (Added an item to the cart), who have not completed a purchase, and have a high likelihood of churning. Then, create a cohort of those users and save it as an audience. Filter the cohort further by likelihood (group), and segment users in the high group, for instance. Another option is to additionally filter by attributed networks and split by the predicted churn event.
Once you have a cohort, export a list of those device IDs or save it as an audience. For more in-depth modeling, tap the Kochava Data Science team as well.
The takeaway
Don’t let the cost of acquiring new users exceed their lifetime value, and stop spending more than your users are. Maximize your ad spend by pre-emptively reengaging those customers most likely to churn before they even think about it leaving.
For more information on how to get started, contacted the Client Success Management team at Kochava.