Measuring true incrementality requires a commitment of time and resources, but advanced A/B testing can determine the effectiveness of campaigns without the same undertaking.
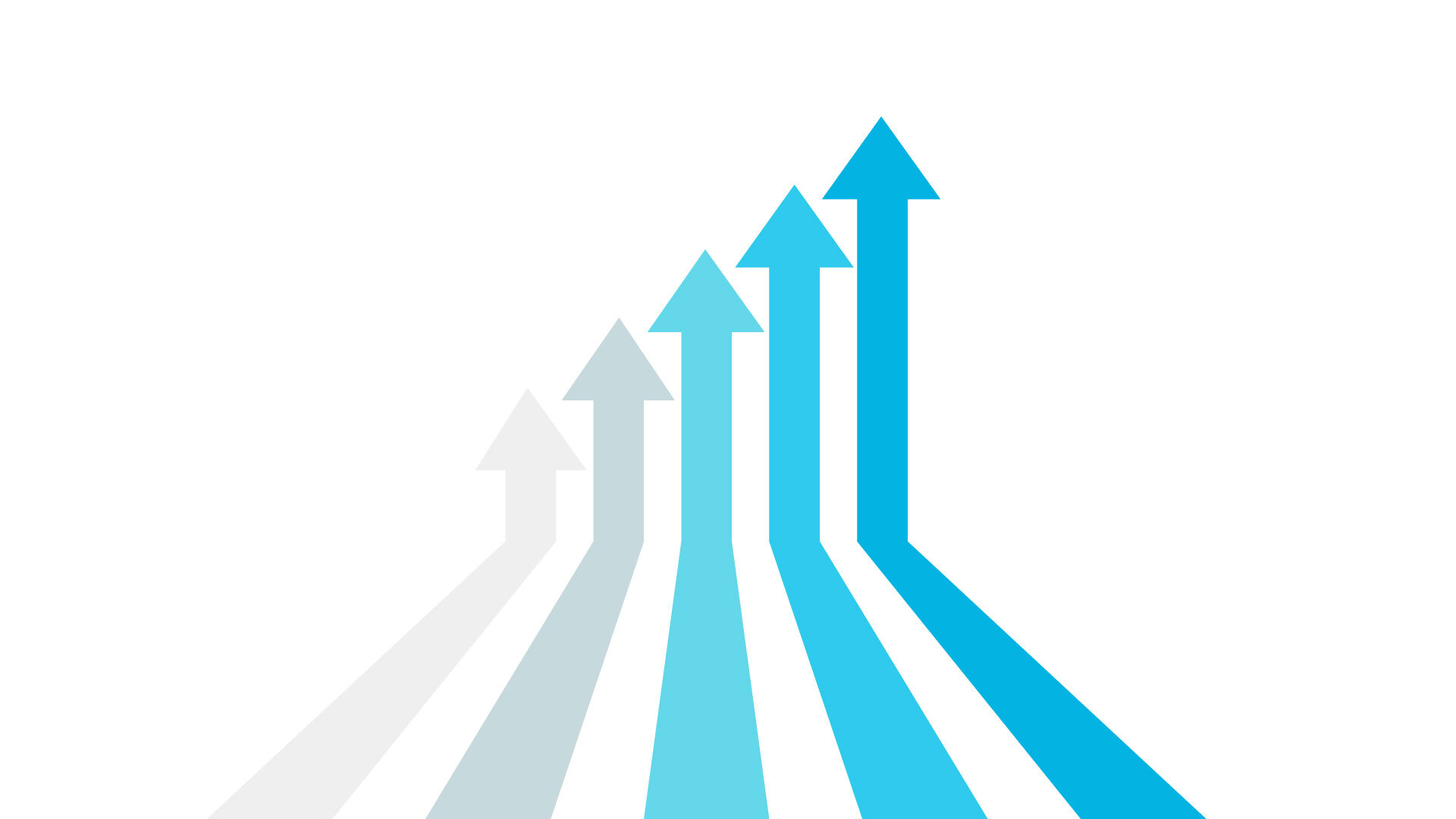
A UA manager runs a campaign for $50K that reached 500K consumers. Of those, 30K installed. Was the campaign successful?
To answer that, you need to know how many people would have purchased in the absence of seeing the ad. This is what incrementality testing promises to answer, but to get it, marketers must endure an often complicated and expensive process. There are other, more affordable avenues that can also answer the question of how effective are your ad campaigns.
The answer—like most things— is “maybe.” But oftentimes, attribution is confused with incrementality. Yet they are very different measurement approaches, and each seeks a different outcome.
Incrementality and lift
Incrementality has become a buzzword of late as marketers want to not simply measure campaign outcomes but determine whether their ads truly influenced conversions. The problem is, what they’re asking for may not be what’s most practical for them as a business. Performing an incrementality exercise requires a commitment of time and money, which also includes an opportunity cost.
What is true incrementality?
To measure incrementality (aka lift or causality), you need to measure the amount of consumers who would have converted (ie, purchased) regardless of whether they saw your ad.
One thing to clear up—performing an incrementality exercise is not the same as attribution. A click does not drive an install, as is commonly discussed in the ecosystem. Too often, we have equated a consumer interaction with an ad with direct correlation to an action (event), but correlation does not equal causality. There are many factors that drive an install, and we’ll never know all of them.
Incrementality testing oftentimes involves segmenting an eligible audience from which you carve out a holdout or control group. This group is suppressed and receives no advertising. You then advertise to the other half and compare conversion rates. This is where incrementality testing starts to get tricky.
From the group that received advertising, you can’t verify that everyone in that group saw the ad. Of those who saw the ad, you still won’t know if some in the group are brand loyalists and would have converted regardless of seeing the ad. Additionally, of those who received your ads, there is the likelihood of data bias since you are competing for the same pool of high quality consumers as other advertisers. Chances are, you will win more bids for lower quality consumers. Lastly, to measure lift, requires multiple tests, which is costly in addition to the opportunity cost lost from not advertising to the holdout group.
Let’s talk about PSAs and ghost ads
When you perform an incrementality test with a holdout group, you can’t compare the outcome of an ad campaign fairly because they haven’t been served any ads. To create a fairer comparison, you can split the holdout group and serve public service announcements (PSAs) or ghost ads (flagged consumers who would have been served an ad) and then compare their behavior. Bids must be the same as the group you advertised to because you want this population to look like the ones who saw your ad.
In spite of the efforts to create an apples-to-apples comparison between the two groups, the groups still look different because with PSAs there is no call to action (CTA). The PSAs act as a placeholder to see if what consumers do after they see a PSA is on par with what those in the advertised group do. However, the two segments won’t have had the same ad experience (as with a CTA).
Possible comparisons
How likely is it that two populations will match?
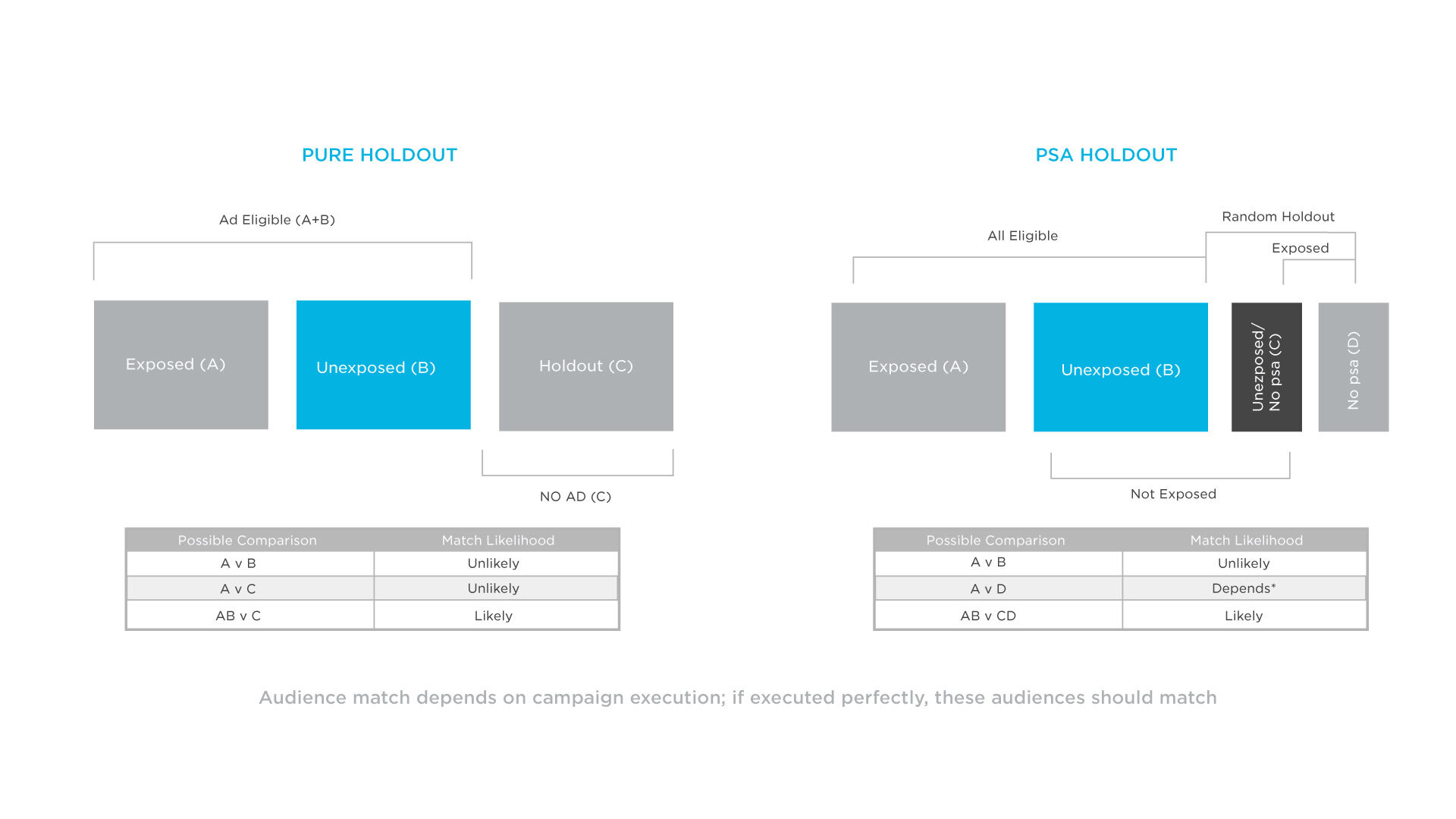
What I’ve outlined above doesn’t paint a pretty picture of incrementality testing. It’s not to say that you can’t do it, but it’s important to lay all the cards on the table and be clear about what it entails since there are some misconceptions about how it works, the perceived value, and the costs. What eventually answers the question of incrementality is time and repetition to create reproducible results and see what factors caused a lift in a campaign.
Viable solutions: A/B testing with a verified data set
In lieu of incrementality, there are a number of more cost-effective alternatives to determine campaign impact through performance.
Going back to the example at the beginning, a UA manager could create an audience of 500K consumers and suppress a segment of those consumers as the holdout group. They could advertise to the other portion and more easily compare the outcomes of that group with the history of the holdout group for say the past 30 days.
Other analysis options include:
- Time series analysis: This type of analysis involves alternately turning marketing off and back on to establish a baseline and to see incremental lifts from networks. Although effective, there is an opportunity cost in turning off all marketing temporarily.
- Comparative market analysis: Analysts define a designated marketing area (DMA) to find geographical pockets that behave similarly. They then surge the marketing in one DMA and refrain from the other. There is a strong chance of seeing conversion rate differences between the two DMAs but also an opportunity cost in surging marketing in one DMA and withholding efforts in the other.
- Time To Install Quality Inference: This analysis compares the time of engagement vs. the quality of user graphs to easily understand what is causal or non-causal. While there is no opportunity cost, this type of analysis is less precise than others.
- Forensic control analysis: This type of analysis is a modeling exercise in which a control group is created that mirrors the exposed group after a campaign has run. The response and performance is weighted up or down based on an algorithm (created from predictive variables). While there is no opportunity cost, copious amounts of data are required to create the model universe.
Most important: Adopt a test & learn mentality
Having a known universe of devices between a group of consumers exposed to ads or not is what’s difficult to obtain with incrementality testing. While incrementality testing is possible, its feasibility is another story. Know your threshold for testing and perhaps consider some of the options outlined above to measure success. Overall, adopting a test-and-learn mentality is what leads to successful marketing.
Interested in learning more? See how our team may help yours through our consulting services.